Animation by matplotlib
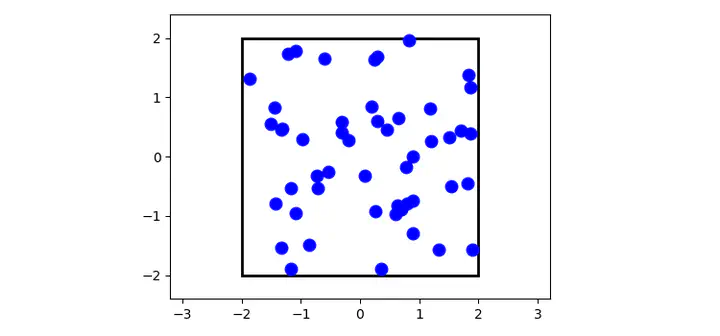
Projects description
Purpose: This is my notes for using Matplotlib to make animation, it’s very convenient during your simulation.
Reference
[1] Official example
[2] Official web
[3] Blog
Basic Animation
Firstly, let’s try some basic case, using the FuncAnimation
tool.
def __init__(self, fig, func, frames=None, init_func=None, fargs=None,
save_count=None, **kwargs):
For FuncAnimation
class, it requires some parameters like,
figure
,the figure object in Matplotlib
func
(callable), it’s a callable function, used to update the object at each frame.
frames
, source of data to pass func
and each frame of the animation
init_func
, A function used to draw a clear frame.
The most important parameters are func
and frames
, so we will introduce them in details.
frames
frames
is the source of data to pass func
and each frame of the animation, and it can be iterable, int, generator function, or None.
- Iterable:
frames=np.linspace(-np.pi,np.pi, 90)
Above is an example of frames
being a list(iterable), ranging from $[-\pi, \pi]$ with 90 frames
- int:
frames=200
This example is from the case1 below, it means there are 200 frames in the final animation.
None: This equivalent to passing
itertools.count
generator function
This is a little complex, for passing a generator as frames
, the generator must be like
def gen_function() -> obj
No input parameters in generator, but one output for passing into func
func
func
is a callable function, used to update the object at each frame. It should be like this:
def func(frame, *fargs) -> iterable_of_artists
So, we always use it to update the figure, such as the following example:
def update(frame):
xdata.append(frame)
ydata.append(np.sin(frame))
ln.set_data(xdata, ydata)
return ln,
Generally
frames
determine the range of frames in animation, it will iterate one time during a interval and then passes the value to func
, until the entire Frames has iterated.
Code case
Case 1: Simple moving $sin$
import itertools
import numpy as np
from matplotlib import pyplot as plt
from matplotlib import animation
# First set up the figure, the axis, and the plot element we want to animate
fig = plt.figure()
ax = plt.axes(xlim=(0, 2), ylim=(-2, 2))
line, = ax.plot([], [], lw=2)
# then, initialization function: plot the background of each frame
def init():
line.set_data([], [])
return line,
# afterwards, set the animation function. This is called sequentially
def animate(i):
x = np.linspace(0, 2, 1000)
y = np.sin(2 * np.pi * (x - 0.01 * i))
line.set_data(x, y)
return line,
anim = animation.FuncAnimation(fig, animate, init_func=init,
frames=None, interval=20, blit=True)
anim.save('./fig/case1.gif', fps=30)
Case 2: Decay
def data_gen():
for cnt in itertools.count():
t = cnt / 10
yield t, np.sin(2*np.pi*t) * np.exp(-t/10.)
def init():
ax.set_ylim(-1.1, 1.1)
ax.set_xlim(0, 10)
del xdata[:]
del ydata[:]
line.set_data(xdata, ydata)
return line,
fig, ax = plt.subplots()
line, = ax.plot([], [], lw=2)
ax.grid()
xdata, ydata = [], []
def run(data):
# update the data
t, y = data
xdata.append(t)
ydata.append(y)
xmin, xmax = ax.get_xlim()
if t >= xmax:
ax.set_xlim(xmin, 2*xmax)
ax.figure.canvas.draw()
line.set_data(xdata, ydata)
return line,
# herein, run is the func, data_gen is the frames
ani = animation.FuncAnimation(fig, run, data_gen, interval=10, init_func=init)
ani.save('./fig/case2.gif', fps=30)
Case 3: Using figure as flame
I don’t recommend you use matplotlib
to make animation based on figures already exits, as the advantage of matplotlib
is the rendering figures.
Instead of matplotlib
, you can use os
and imageio
to make animation based on figures.
import os
import imageio
def create_gif(image_list, gif_name):
frames = []
for image_name in image_list:
if image_name.endswith('.tif'):
print(image_name)
frames.append(imageio.imread(image_name))
# Save them as frames into a gif
imageio.mimsave(gif_name, frames, 'GIF', duration = 0.1)
return
def main():
path=r'./fig/frames/'
image_list=[ path+img for img in os.listdir(path)]
gif_name = os.listdir(path)[-1][:-4]+'.gif'
create_gif(image_list, gif_name)
if __name__ == "__main__":
main()
Case 4 : using colorbar
In this case, we try to add colorbar to a gif. It’s kind of tricky, as it may be
import numpy as np
import matplotlib.pyplot as plt
import matplotlib.animation as animation
from mpl_toolkits.axes_grid1 import make_axes_locatable
plt.rcParams["figure.figsize"] = [7.50, 3.50]
plt.rcParams["figure.autolayout"] = True
fig = plt.figure()
ax = fig.add_subplot(111)
div = make_axes_locatable(ax)
cax = div.append_axes('right', '5%', '5%')
data = np.random.rand(5, 5)
im = ax.imshow(data)
cb = fig.colorbar(im, cax=cax)
tx = ax.set_title('Frame 0')
cmap = ["copper", 'RdBu_r', 'Oranges', 'cividis', 'hot', 'plasma']
def animate(i):
cax.cla()
data = np.random.rand(5, 5)
im = ax.imshow(data, cmap=cmap[i%len(cmap)])
fig.colorbar(im, cax=cax)
tx.set_text('Frame {0}'.format(i))
ani = animation.FuncAnimation(fig, animate, frames=10)
ani.save('./fig/case5.gif', fps=30)
Case 5: Raindrops
This case is more complexed than above. Firstly, we should generate the data, then use animation to render those data.
# Fixing random state for reproducibility
np.random.seed(19680801)
# Create new Figure and an Axes which fills it.
fig = plt.figure(figsize=(7, 7))
ax = fig.add_axes([0, 0, 1, 1], frameon=False)
ax.set_xlim(0, 1), ax.set_xticks([])
ax.set_ylim(0, 1), ax.set_yticks([])
# Create rain data
n_drops = 50
rain_drops = np.zeros(n_drops, dtype=[('position', float, (2,)),
('size', float),
('growth', float),
('color', float, (4,))])
# Initialize the raindrops in random positions and with
# random growth rates.
rain_drops['position'] = np.random.uniform(0, 1, (n_drops, 2))
rain_drops['growth'] = np.random.uniform(50, 200, n_drops)
# Construct the scatter which we will update during animation
# as the raindrops develop.
scat = ax.scatter(rain_drops['position'][:, 0], rain_drops['position'][:, 1],
s=rain_drops['size'], lw=0.5, edgecolors=rain_drops['color'],
facecolors='none')
def update(frame_number):
# Get an index which we can use to re-spawn the oldest raindrop.
current_index = frame_number % n_drops
# Make all colors more transparent as time progresses.
rain_drops['color'][:, 3] -= 1.0/len(rain_drops)
rain_drops['color'][:, 3] = np.clip(rain_drops['color'][:, 3], 0, 1)
# Make all circles bigger.
rain_drops['size'] += rain_drops['growth']
# Pick a new position for oldest rain drop, resetting its size,
# color and growth factor.
rain_drops['position'][current_index] = np.random.uniform(0, 1, 2)
rain_drops['size'][current_index] = 5
rain_drops['color'][current_index] = (0, 0, 0, 1)
rain_drops['growth'][current_index] = np.random.uniform(50, 200)
# Update the scatter collection, with the new colors, sizes and positions.
scat.set_edgecolors(rain_drops['color'])
scat.set_sizes(rain_drops['size'])
scat.set_offsets(rain_drops['position'])
# Construct the animation, using the update function as the animation director.
ani = animation.FuncAnimation(fig, update, interval=10)
ani.save('./fig/case6.gif', fps=30)
Case 6: Particles in box $^{[3]}$
This is also a simulation case, so, remember to separate data generation and animation.
This code simulates the elastic collisions of a group of particles in a box under the force of gravity. The collisions are elastic: they conserve energy and 2D momentum, and the particles bounce realistically off the walls of the box and fall under the influence of a constant gravitational force.
import scipy.integrate as integrate
from scipy.spatial.distance import pdist, squareform
class ParticleBox:
"""Orbits class
init_state is an [N x 4] array, where N is the number of particles:
[[x1, y1, vx1, vy1],
[x2, y2, vx2, vy2],
... ]
bounds is the size of the box: [xmin, xmax, ymin, ymax]
"""
def __init__(self,
init_state = [[1, 0, 0, -1],
[-0.5, 0.5, 0.5, 0.5],
[-0.5, -0.5, -0.5, 0.5]],
bounds = [-2, 2, -2, 2],
size = 0.04,
M = 0.05,
G = 9.8):
self.init_state = np.asarray(init_state, dtype=float)
self.M = M * np.ones(self.init_state.shape[0])
self.size = size
self.state = self.init_state.copy()
self.time_elapsed = 0
self.bounds = bounds
self.G = G
def step(self, dt):
"""step once by dt seconds"""
self.time_elapsed += dt
# update positions
self.state[:, :2] += dt * self.state[:, 2:]
# find pairs of particles undergoing a collision
D = squareform(pdist(self.state[:, :2]))
ind1, ind2 = np.where(D < 2 * self.size)
unique = (ind1 < ind2)
ind1 = ind1[unique]
ind2 = ind2[unique]
# update velocities of colliding pairs
for i1, i2 in zip(ind1, ind2):
# mass
m1 = self.M[i1]
m2 = self.M[i2]
# location vector
r1 = self.state[i1, :2]
r2 = self.state[i2, :2]
# velocity vector
v1 = self.state[i1, 2:]
v2 = self.state[i2, 2:]
# relative location & velocity vectors
r_rel = r1 - r2
v_rel = v1 - v2
# momentum vector of the center of mass
v_cm = (m1 * v1 + m2 * v2) / (m1 + m2)
# collisions of spheres reflect v_rel over r_rel
rr_rel = np.dot(r_rel, r_rel)
vr_rel = np.dot(v_rel, r_rel)
v_rel = 2 * r_rel * vr_rel / rr_rel - v_rel
# assign new velocities
self.state[i1, 2:] = v_cm + v_rel * m2 / (m1 + m2)
self.state[i2, 2:] = v_cm - v_rel * m1 / (m1 + m2)
# check for crossing boundary
crossed_x1 = (self.state[:, 0] < self.bounds[0] + self.size)
crossed_x2 = (self.state[:, 0] > self.bounds[1] - self.size)
crossed_y1 = (self.state[:, 1] < self.bounds[2] + self.size)
crossed_y2 = (self.state[:, 1] > self.bounds[3] - self.size)
self.state[crossed_x1, 0] = self.bounds[0] + self.size
self.state[crossed_x2, 0] = self.bounds[1] - self.size
self.state[crossed_y1, 1] = self.bounds[2] + self.size
self.state[crossed_y2, 1] = self.bounds[3] - self.size
self.state[crossed_x1 | crossed_x2, 2] *= -1
self.state[crossed_y1 | crossed_y2, 3] *= -1
# add gravity
self.state[:, 3] -= self.M * self.G * dt
#------------------------------------------------------------
# set up initial state
np.random.seed(0)
init_state = -0.5 + np.random.random((50, 4))
init_state[:, :2] *= 3.9
box = ParticleBox(init_state, size=0.04)
dt = 1. / 30 # 30fps
#------------------------------------------------------------
# set up figure and animation
fig = plt.figure()
fig.subplots_adjust(left=0, right=1, bottom=0, top=1)
ax = fig.add_subplot(111, aspect='equal', autoscale_on=False,
xlim=(-3.2, 3.2), ylim=(-2.4, 2.4))
# particles holds the locations of the particles
particles, = ax.plot([], [], 'bo', ms=6)
# rect is the box edge
rect = plt.Rectangle(box.bounds[::2],
box.bounds[1] - box.bounds[0],
box.bounds[3] - box.bounds[2],
ec='none', lw=2, fc='none')
ax.add_patch(rect)
def init():
"""initialize animation"""
global box, rect
particles.set_data([], [])
rect.set_edgecolor('none')
return particles, rect
def animate(i):
"""perform animation step"""
global box, rect, dt, ax, fig
box.step(dt)
ms = int(fig.dpi * 2 * box.size * fig.get_figwidth()
/ np.diff(ax.get_xbound())[0])
# update pieces of the animation
rect.set_edgecolor('k')
particles.set_data(box.state[:, 0], box.state[:, 1])
particles.set_markersize(ms)
return particles, rect
ani = animation.FuncAnimation(fig, animate, frames=600,
interval=10, blit=True, init_func=init)
ani.save('./fig/case7.gif', fps=30)
#ani.save('particle_box.mp4', fps=30, extra_args=['-vcodec', 'libx264'])